In recent years, organizations have strategically embraced cloud migration to attain agility and scalability. The cloud's innovation has redefined data storage and accessibility, but this is only the beginning of what's possible. Storing data in the cloud without extracting insights is akin to possessing a treasure chest that remains unopened. Countless organizations have amassed data, yet the enormous potential of this information remains largely untapped. The real challenge is transforming this raw data into actionable intelligence that fuels decision-making and innovation. The solution to this challenge is data mining, which is, in essence, the alchemy of converting data into gold.
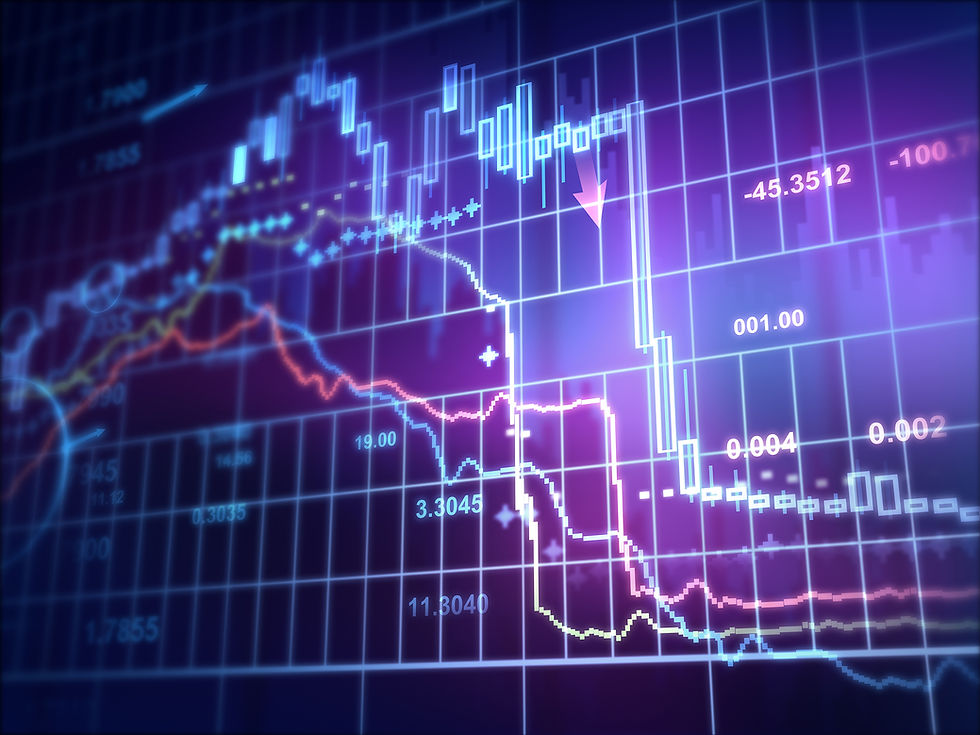
Turning Data into Gold
Data mining, alternatively termed as Knowledge Discovery in Data (KDD), is the systematic technique for extracting noteworthy patterns and useful information from expansive data sets. This process, which draws upon principles from statistics, artificial intelligence, and machine learning, has become an essential tool in our current era, characterized by an explosion in data generation. It involves various techniques such as clustering (grouping related items together, like k-means or hierarchical clustering), classification (predicting the category of an item, like decision trees or support vector machines), and association rule learning (discovering interesting relations between variables, like the Apriori algorithm).
As an automated or semi-automated technical process, data mining sifts through vast sets of data to unveil meaningful patterns, correlations, and insights, empowering organizations to pivot and decide with agility and precision.
In the current landscape, data is proliferating at a breakneck speed. Astoundingly, 90% of the world's data has been generated since 2014, and the sheer volume of data is doubling approximately every 1.2 years [1]. This rapid data expansion necessitates an adept navigator, and data mining serves as the astrolabe guiding companies through the sea of information. Through analytics and data mining, businesses can meticulously craft models that uncover interconnections among millions of data points.
Data Mining for Actionable Insights
In today's fiercely competitive business environment, data-driven decision-making is critical. It enables organizations to formulate strategic decisions based on robust, actionable evidence, which ultimately boosts their operational efficiency and provides them with a competitive edge. A PwC survey involving over 1,000 high-ranking executives demonstrated that organizations that heavily rely on data are three times more likely to experience significant improvements in their decision-making process compared to those less dependent on data [1].
What makes data mining powerful is its ability to uncover hidden relationships and patterns in data that human analysts or other analysis techniques may overlook. Given that around 80% of an organization's data is unstructured, data mining plays a critical role in deciphering these untapped insights. These insights can assist businesses in making more informed decisions, thereby potentially increasing revenue or optimizing marketing efforts. However, it's crucial to note that data mining uncovers patterns, not causal relationships, and hence doesn't replace the need for skilled analysts who understand the business, the data, and the techniques and processes of data mining [2].
Data mining is revolutionizing various business functions. Here’s a snapshot of how different sectors are harnessing the power of data mining.
Accelerate Product Innovations
While Customer Relationship Management Systems (CRM) are integral to managing customer feedback, many companies still grapple with leveraging this data to address service issues or spark innovation. This is where the power of data mining comes to the rescue. The application of data mining techniques to analyze customer feedback is a vibrant research domain, with various models proposed, such as cluster analysis and predictive modeling, to identify and tackle challenges.
Many companies have practically harnessed the potential of data mining in their innovation strategies, gaining significant insights from vast customer feedback to steer product development and marketing efforts. Consider the example of a global home appliance leader that manages an enormous monthly data influx, comprising around a million reviews from 5,000 to 10,000 customers, sourced from 40 websites and six different platforms, including emails and sales representative contact forms.
This company employs sophisticated data mining software to sift through this enormous data to understand product performance, customer preferences, purchasing experiences, and market competitiveness. This data analysis fosters a shared language and facilitates comprehensive competitive product analysis.
The outcomes of this data-centric approach are remarkable, condensing the innovation cycle from a 24–28-month timeline to just 11-12 months. Additionally, it reveals insightful customer value patterns; for instance, the company discovered that 40% of customers appreciate energy efficiency, 35% prioritize lower carbon footprints, 32% are attracted to cost-effective products, and 26% value time-saving innovations. This knowledge enables the creation of innovations that resonate with the consumers' everyday needs and preferences [3] [4].
Better recommendation to customers
In an era of boundless choices, recommender systems, powered by data mining techniques, aid users in discovering and evaluating items that suit their preferences. These systems develop user profiles by studying user actions and attributes over time, employing techniques such as clustering, classification, association rules generation, and the creation of similarity graphs like Horting.
A prominent example of data mining in recommender systems is the use of association rules or item-to-item correlations. This method identifies items commonly associated with a user's interests, using factors like co-purchase data and preferences shared by other users. For instance, this approach can identify products that often pair well with a selected item, improving cross-selling and upselling opportunities. It may also allow merchants to strategically arrange products so that related items, like batteries for a handheld video game, are easily accessible. Advanced temporal data mining can even predict future purchases based on current buying behavior.
From renowned platforms like Netflix and Amazon to smaller eCommerce sites, this technology is widely utilized, offering personalized recommendations to enhance the user experience and drive sales.
Beware of Pitfalls
Following cloud migration, integrating data mining techniques demands a tactical approach. Selecting tools and solutions that resonate with the business objectives is imperative. Furthermore, data mining calls for an amalgamation of skills spanning big data, computing, and information analysis. Given the skyrocketing demand for data professionals, as evidenced by the U.S. Bureau of Labor Statistics prediction that data science would experience higher growth than most fields from now to 2029 [5], it’s pivotal to be vigilant about the challenges ensnaring the path of data mining implementation:
Scattered Data Landscape: Data strewn across multiple platforms, such as databases, personal systems, or the web, impedes centralization. With regional offices often hosting their data servers, pooling all data in a central repository is a Sisyphean task. Thus, developing bespoke tools and algorithms for mining scattered data is essential.
Data Complexity: Handling high volumes of diverse data, including structured, semi-structured, unstructured, and multimedia forms, can be costly and time-consuming. Utilizing advanced data mining techniques such as clustering, classification, and association rule mining helps identify patterns and relationships for insight extraction and predictions.
Incomplete and Noisy Data: Large datasets are often marred by inaccuracies or unreliability due to equipment glitches. Incomplete data and system-induced noise obstruct the data mining process. Implementing effective data cleaning and preprocessing methods helps to address these data issues. Such advanced techniques as K-Nearest Neighbors (KNN) for the imputation of missing values or Robust Scaler to handle outliers can be taken into consideration.
Security and Privacy Dilemma: With data exchange crucial to decision-making, safeguarding against unauthorized access, especially when dealing with sensitive personal information, is critical. Practitioners should adopt data anonymization and encryption techniques to maintain privacy and data security, removing personally identifiable information (PII) and encoding data to make it unreadable to unauthorized users.
Budgetary Constraints: Financial barriers can hinder the acquisition and upkeep of powerful servers, software, and hardware required to manage extensive data quantities. To navigate these budget restrictions, consider options like cost-effective solutions, utilizing pay-as-you-go services from reputable providers, and prioritizing expenditures based on business necessities.
Conclusion: The Golden Key
In a nutshell, data mining serves as the golden key for organizations seeking to unlock the treasure trove of cloud data. Through its applications across industries such as Telecommunications, Retail and E-commerce, Healthcare, and Media and Entertainment, data mining has proven to be indispensable in uncovering actionable insights. However, the path to successfully utilizing data mining is riddled with challenges, including data complexity, security concerns, and the need for domain expertise.
As the linchpin in transforming raw data into strategic assets, organizations must adeptly navigate these challenges by aligning their data mining efforts with their business objectives, investing in the right tools, and fostering a culture of data-driven decision-making.
Source: FPT Software
Comments